Automate Your Data Management: How AI Agents Revolutionize Validation
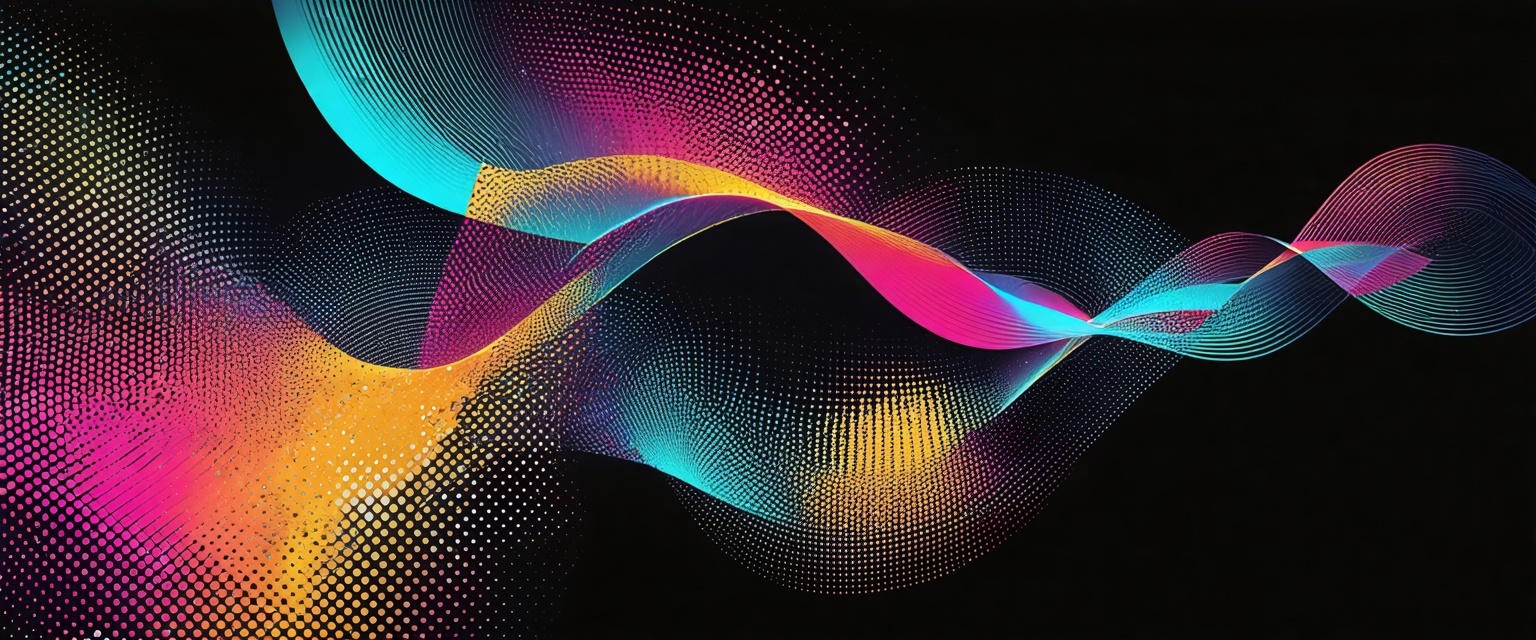
Discover how to streamline data management using AI agents for precise validation, reducing errors and boosting productivity. Elevate your data strategy now!
Is your business overwhelmed by the challenge of integrating data from multiple sources? Are manual data processes causing delays and errors that hinder your productivity? Learning how to automate data validation using AI agents can help overcome the complexities of managing diverse data sets, improving decision-making, and accelerating your operations.
By incorporating AI agents for data validation, Datagrid's data connectors provide a targeted solution to these problems by seamlessly linking your various data sources.
In this article, we'll explore how you can leverage Datagrid's data connectors and agentic AI to optimize your data handling workflows.
How to Use AI Agents for Data Validation
Overview of Data Validation
Data validation ensures your business data is accurate, consistent, and complete. Reliable data forms the foundation of effective decision-making across tasks like machine learning models, analytics reports, and business intelligence dashboards. Validating your data prevents errors and avoids the costly process of data cleansing, maintaining the standards essential for operational efficiency.
With data coming from multiple sources and needing quick action, validation is an ongoing process that guards against inconsistencies and preserves data integrity. Techniques like type checks, range checks, and format verifications help maintain consistency across diverse datasets, allowing for seamless integration across different platforms. In essence, robust data validation is vital for making informed decisions based on trustworthy information.
Relevance of AI in Data Validation
AI agents simplify data validation by automating repetitive tasks, reducing human error, and speeding up workflows. By integrating AI to automate data integration, businesses can process large datasets more efficiently. AI systems learn from historical data patterns to detect anomalies, fill in missing information, and ensure data aligns with predefined standards.
As these systems evolve with continuous human feedback, they improve their precision in handling validation tasks. Each interaction enhances data quality, helping businesses achieve more reliable datasets for decision-making.
This reduces the manual workload on your team, allowing them to focus on higher-level problem-solving and planning. Adopting AI-driven solutions helps maintain data integrity and reliability, leading to better outcomes for your organization.
Understanding Data Validation
What is Data Validation?
Data validation is about ensuring that your data is accurate, consistent, and reliable. It involves checking data against set rules to determine if it's suitable for analysis and decision-making. In a world driven by data, precision matters because the insights gained are only as good as the data they're based on.
Validation checks are applied at various stages to prevent errors, redundancies, or inconsistencies. Whether during data entry or batch processing, these checks help identify issues like incorrect formats or duplication. Real-time validation ensures data integrity at the point of entry, confirming that entries meet acceptable criteria.
This process is vital because validated data supports reliable analyses and business activities. It not only improves data quality but also saves time and resources by reducing the need for extensive data cleansing later on.
The Role of AI Agents in Data Validation
AI agents are transforming data validation by introducing automation and intelligence to the process. Using machine learning, AI streamlines validation workflows, making them more efficient. AI systems can identify patterns, detect anomalies, and adapt to changing data environments, ensuring data validity and integrity are maintained.
These agents learn from each validation task, gradually improving their accuracy and efficiency. With each round of checks, they refine their processes, reducing errors and the need for human intervention. This adaptability is crucial when managing large and dynamic datasets, where the data flow can be overwhelming.
AI can also be used to automate lead enrichment with AI, enhancing the quality of customer data for better engagement and sales strategies.
Moreover, AI-driven validation tools can perform checks in real-time, providing timely insights critical for swift decision-making. By automating tedious tasks, AI agents allow faster data processing and validation, leading to cost savings and improved workflow efficiency. Combining AI with traditional validation techniques not only enhances data quality but also empowers organizations to make more informed decisions based on reliable data.
Types of Data Suitable for AI Validation
AI agents excel at validating various types of data, enhancing reliability and utility across multiple sectors.
Types of Data
- Structured Data: Organized data like that in databases and spreadsheets. AI validation ensures consistency, accuracy, and correct formatting, quickly verifying structured data to maintain integrity.
- Unstructured Data: Includes text, images, audio, and video without a predefined model. AI uses natural language processing and computer vision to extract insights and validate these formats. For example, you can data mine a PDF to extract valuable information from documents.
- Semi-Structured Data: Formats like JSON and XML. AI agents parse and validate this data to ensure it adheres to expected schemas and formats.
- Time-Series Data: Data collected over time, such as stock prices or sensor outputs. AI analyzes trends and detects anomalies, crucial for ensuring data continuity and accuracy.
- Geospatial Data: Used in mapping and navigation. AI validates geospatial data by confirming accurate coordinates and alignment with maps and landmarks.
- Sensor Data: Found in IoT applications. AI agents validate real-time sensor data, using statistical models to ensure integrity from continuous streams.
Integration with Tools and SystemsAI validation systems become even more powerful when integrated with various tools and systems:
- API Integration: AI agents interact with web services to fetch real-time data, maintaining consistency and preventing discrepancies.
- Cloud-Based Platforms: Using platforms like AWS or Azure, AI validation can handle scalable tasks for large datasets.
- Database Management Systems: Integration with systems like SQL Server allows AI agents to validate data directly within databases.
- Big Data Platforms: Working with technologies like Hadoop and Spark, AI tools process and validate massive datasets efficiently.
- Business Intelligence Tools: AI validation enhances data accuracy within BI tools, supporting data-driven decisions.
- Data Management Platforms: Automating validation tasks within data management solutions, such as when you connect Salesforce with Google Sheets, ensures seamless data flow and error prevention.
These integrations enable robust validation processes, helping organizations maintain accurate data and make strategic decisions. For instance, you can connect Salesforce with PandaDoc to streamline document workflows and enhance data consistency.
Benefits of AI Agents in Data Validation
Efficiency and Accuracy
AI agents play a crucial role in boosting the efficiency and accuracy of data validation. By automating data entry with AI and other repetitive tasks, they free up your team to focus on strategic, higher-value activities. While the initial setup can be intensive, integrating AI streamlines future data additions, saving time and resources.
AI systems are adept at identifying errors and inconsistencies across large datasets with precision. They use advanced algorithms to detect patterns and anomalies that might slip past human analysts.
This ensures that your data analyses are built on a solid foundation, eliminating potential inaccuracies. Additionally, AI can harmonize data from multiple sources, ensuring cross-compatibility, which is essential for comprehensive analysis.
Moreover, AI agents improve through continuous learning. Each human correction helps the AI system refine its processes, reducing the workload over time. This self-improving capability not only enhances accuracy but also speeds up validation as the system adapts and becomes more reliable.
Another advantage is that AI can operate around the clock without fatigue, ensuring ongoing processes and timely error detection. Prompt identification and correction of errors maintain the consistent data quality necessary for effective decision-making and operational efficiency.
Scalability and Cost-Effectiveness
AI agents offer unmatched scalability in data validation. As your data grows, AI systems scale effortlessly, handling increased workloads without sacrificing performance. Traditional methods might struggle under heavy loads, but AI dynamically allocates resources to prevent bottlenecks, supporting seamless operation even as data volumes change.
Cost-effectiveness is another key benefit. While there's an initial investment in AI technologies, the reduction in labor costs and improvement in data accuracy offset these expenses. Companies can choose between open-source solutions or commercial APIs, offering financial flexibility to fit their budge.
Using AI for data validation leads to reduced error rates, increased productivity, and the ability to integrate new data sources quickly—all without significant extra costs. This makes AI a smart, cost-efficient strategy for organizations looking to enhance operational efficiency.
Industry Applications
AI-driven data validation is making a significant impact across various sectors, streamlining operations and improving data accuracy. For example, organizations can automate claims comparison to enhance efficiency and reduce errors. Let's look at how different industries are leveraging these advancements.
Healthcare
In healthcare, AI-driven data validation is vital for maintaining accurate patient records, aiding diagnostics, and optimizing resource allocation. AI ensures data consistency across systems, reducing errors in patient records and improving outcomes.
By validating patient data and even diagnostic images, AI supports early diagnosis and personalized treatment planning. This not only enhances patient care but also improves administrative efficiency by automating tasks like record updates and claims forms extraction, leading to faster claims processing.
Finance
The finance industry relies on precise data for trading, risk management, and compliance. AI-driven data validation ensures data integrity, preventing fraud and minimizing transaction errors. AI tools can verify large volumes of data in real time, optimizing back-office operations and strengthening compliance efforts. This leads to better decision-making and reduced operational risk.
Manufacturing
Manufacturers use AI validation for predictive maintenance, quality control, and supply chain optimization. AI analyzes data from machinery sensors to schedule timely maintenance, minimizing downtime. On production lines, AI detects defects, ensuring consistent quality and reducing waste. By examining supply chain data, AI identifies bottlenecks and optimizes logistics, boosting efficiency.
Retail
In retail, accurate data across channels is crucial for inventory management and personalized marketing. AI validation helps manage sales transactions, stock levels, and customer data, improving demand forecasting and inventory decisions. Validated customer data allows for tailored marketing strategies, enhancing engagement and satisfaction.
Telecommunications
In telecommunications, data validation ensures accurate customer profiles and efficient service delivery. AI systems manage vast amounts of customer data, ensuring consistency and reducing errors in billing and network management. This optimization enhances customer satisfaction and reduces costs.
Across these industries, AI-driven data validation enhances data reliability, supports strategic decisions, and improves operational efficiency. As AI evolves, its role in transforming industry practices will continue to grow, setting new standards for data management.
Challenges and Limitations
While AI agents significantly improve data validation, they also present challenges and limitations that need careful attention.
Potential Challenges
One major challenge is dealing with complex data sources. Data comes from diverse origins with varying formats and structures, making consistent validation a tough task. This can lead to integration issues and quality problems during data transformation.
Data quality poses another challenge. AI agents must handle issues like missing, inaccurate, or duplicated data, often resulting from manual entry errors or system inconsistencies. Poor data quality can affect AI performance, leading to unreliable validation results.
Data dynamism adds complexity. With data constantly evolving, AI systems need frequent updates to stay accurate.
Integrating AI into existing infrastructures can be difficult, especially when dealing with large-scale data migration. Solutions that automate insurance data migration can help mitigate these challenges.
Many organizations have legacy systems that aren't compatible with modern AI technologies, potentially causing disruptions or requiring costly upgrades. Additionally, AI systems require large amounts of high-quality data, and inconsistent datasets can lead to inadequate results.
There's also a shortage of skilled personnel in AI, making it hard for organizations to find experts who can manage both AI and data validation processes.
Addressing Limitations
To overcome these challenges:
- Develop Adaptive AI Models: Use AI models that can handle diverse data formats to reduce integration issues. Advanced data cleaning techniques can enhance quality by correcting inaccuracies and filling gaps.
- Implement Robust Data Governance: Establish frameworks that manage diverse data formats. Advanced mapping techniques can maintain consistency and streamline validation.
- Invest in Skilled Personnel and Training: Focus on upskilling existing staff and hiring new talent with expertise in AI and data validation. Training programs can bridge skill gaps.
- Adopt a Hybrid Validation Approach: Combine AI-driven validation with manual reviews to ensure thorough checking. This helps catch errors that AI might miss.
- Integrate Machine Learning Algorithms: Use self-learning models that adjust validation rules as data changes, keeping AI agents effective over time.
- Implement Data Privacy Measures: Use anonymization and encryption to protect privacy and meet regulatory requirements.
By focusing on these strategies, organizations can tackle the challenges of using AI in data validation, ensuring systems are robust, adaptable, and compliant with industry standards.
Implementing AI Agents for Data Validation
Practical Steps to Implementation
Integrating AI agents into your data validation processes, such as when you automate insurance workflows, requires a structured approach:
- Define Clear Objectives: Set specific goals for what you want to achieve with AI-driven validation, such as improving accuracy or speeding up processes.
- Select Appropriate AI Tools and Technologies: Choose AI agents that fit your objectives and can handle your data's complexity. Ensure they integrate smoothly with your current systems.
- Integration with Current Systems: Confirm that the AI agents are compatible with your existing infrastructure to avoid disruptions.
- Develop a Comprehensive Plan: Outline a detailed strategy that includes scope, timelines, resources, and risk management.
- Focus on Security, Privacy, and Compliance: Establish data governance with strong security measures and compliance with regulations like GDPR or HIPAA.
- Human Oversight: Include human review for critical outputs to catch errors and make informed decisions based on AI results.
Pilot Projects and Testing
Before full deployment, pilot projects help assess performance and refine strategies:
- Initiate Controlled Pilots: Start with small-scale deployments to test effectiveness and identify issues.
- Monitor and Evaluate Performance: Use monitoring tools to track AI performance and make timely adjustments.
- Iterative Testing and Feedback: Continuously test and incorporate user feedback to enhance the system.
- Gradual Scaling: Expand the use of AI agents gradually, allowing for controlled scaling and adjustments.
By following these steps and utilizing pilot projects, organizations can integrate AI agents into data validation effectively, ensuring robust and trustworthy processes.
How Agentic AI Simplifies Task Automation
Agentic AI enhances task automation by autonomously solving problems within workflows. By integrating with Datagrid's data connectors and AI agents, professionals can focus on strategic activities while AI handles routine tasks efficiently.
Datagrid connects with over 100 data platforms, including CRM systems like Salesforce, HubSpot, and Microsoft Dynamics 365, ensuring that customer information and sales data are always current.
Agentic AI interprets tasks and executes actions with minimal human oversight. This capability lets businesses use AI for tasks ranging from customer service automation to financial portfolio management, improving efficiency and reducing costs. It enhances customer experiences through personalized interactions and quick responses, freeing professionals to concentrate on innovation and growth.
The architecture of agentic AI includes advanced frameworks and AI agent architectures that support task automation, contributing to efficient decision-making. By utilizing features like AI augmentation, professionals can automate repetitive tasks and focus on strategic initiatives. Ethical considerations in these workflows ensure that AI-driven tasks align with organizational values.
This integration across platforms and focus on efficiency makes agentic AI a vital element in modern task automation.
Simplify Data Validation: How to Use AI Agents
Don't let data complexity slow you down. By learning how to use AI agents for data validation, you can streamline data tasks and boost process efficiency. Datagrid's AI-powered platform is designed for insurance professionals to automate insurance data management, reducing manual processing time and enhancing productivity. This efficient data handling provides instant actionable insights, allowing you to focus on more strategic activities.
With Agentic AI, the platform models the logic of your data validation processes, overcoming technical barriers. Reducing unnecessary data inputs and implementing AI-driven automation decreases operational expenses and increases data accuracy, improving team productivity. See how Datagrid can elevate your team's productivity…